On October 12, a HUST interdisciplinary team, members including Academician Ding Han, Professor Huang Yunhui, and Professor Yuan Ye published a paper entitled "Lithium Battery Online Personalized Health Assessment Based on Deep Transfer Learning"; in the Royal Society of Chemistry's flagship journal, Energy & Environmental Science. The paper was also selected as the cover article. Ma Guijun, a 2017 doctoral student at the Innovation Institute and the School of Mechanical Science and Engineering of HUST, is the first author. He represents the HUST's efforts in cross-disciplinary education.
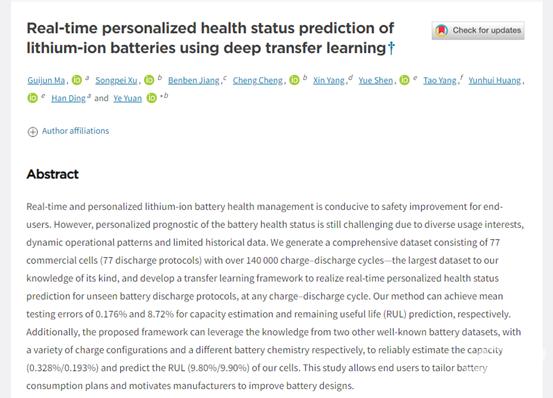

In the context of the carbon peaking and carbon neutrality national goals, the electrification of power systems is an inevitable trend. Health assessment of battery, the "heart of power", is widely acknowledged as a high-value scientific research topic. Battery health assessment technology combined with battery material restoration and recycling technology can optimize energy yield and support the implementation of national goals. In recent years, new battery health assessment methods enabled by machine learning are able to evaluate the cycle life, health status, and remaining effective life of a battery in a specific charge/discharge cycle using a small amount of measurement data. However, for actual scenarios, the current technology has the following two common problems:
(1)Users have different habits of using batteries, and the inconsistency of discharge strategies leads to major contrast among battery data. Building models using data of a specific battery to directly evaluate a battery of different discharge strategy will cause mistakes;
(2)Due to serious reliance on historical data, current technology cannot achieve the evaluation of battery health of real-time batteries which are charged and discharged randomly.
Therefore, existing technology fails to establish a reliable universal model to check the health status of different batteries in real time. The industry urgently needs personalized health management solutions to ensure users' safety and provide scientific reference for manufacturers to improve battery production by providing common and programmable battery usage strategies.
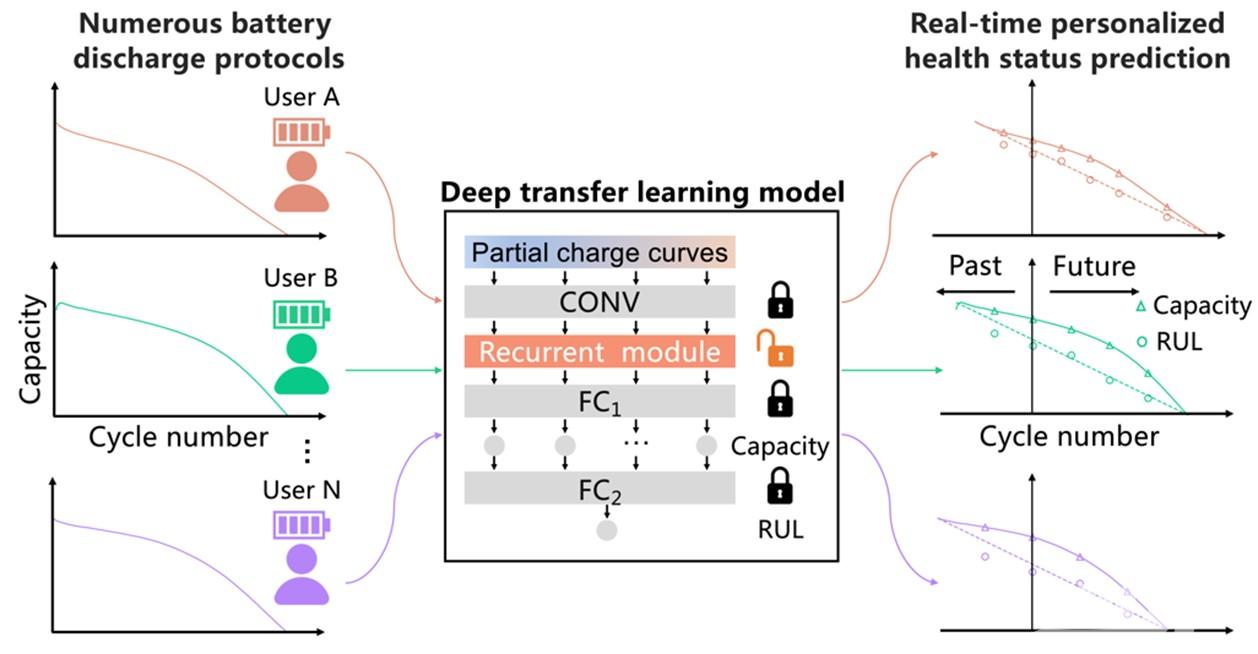
Assess battery health online for different users
Academician Ding Han's team has long been committed to solutions of common scientific problems with interdisciplinary research on machinery, materials, and artificial intelligence. In the face of the battery health assessment challenge, the team innovatively introduced the idea of migration learning in artificial intelligence and customized a real-time battery health assessment plan for users. Using only 30 historical cycle data, this method can realize the health assessment migration between different discharge strategies for batteries of the same material type and the health assessment migration between lithium batteries of different material types. All predictions are completed online. Compared to the general framework proposed by the team in the paper "A General End-to-End Diagnostic Framework for Manufacturing Systems"; published in the National Science Review magazine in 2020, the accuracy of personalized battery health assessments has been increased 3 to 5 times. Compared to the battery health assessment method proposed by the Massachusetts Institute of Technology and Stanford University in the journal Nature Energy, HUST's method not only realizes real-time health assessment customized for different users, but also uses 2/3 less historical data. In addition, the article published the world's largest benchmark dataset of multi-discharge strategy lithium battery to date, which can provide a data benchmark for research in this field. The "real-time personalization"; migration method proposed in the article provides inspirations for battery health management. This method can be adapted to health assessments of other type of battery, and is of great significance to battery manufacturing, testing, and recycling.
Related links: https://pubs.rsc.org/en/content/articlelanding/2022/ee/d2ee01676a